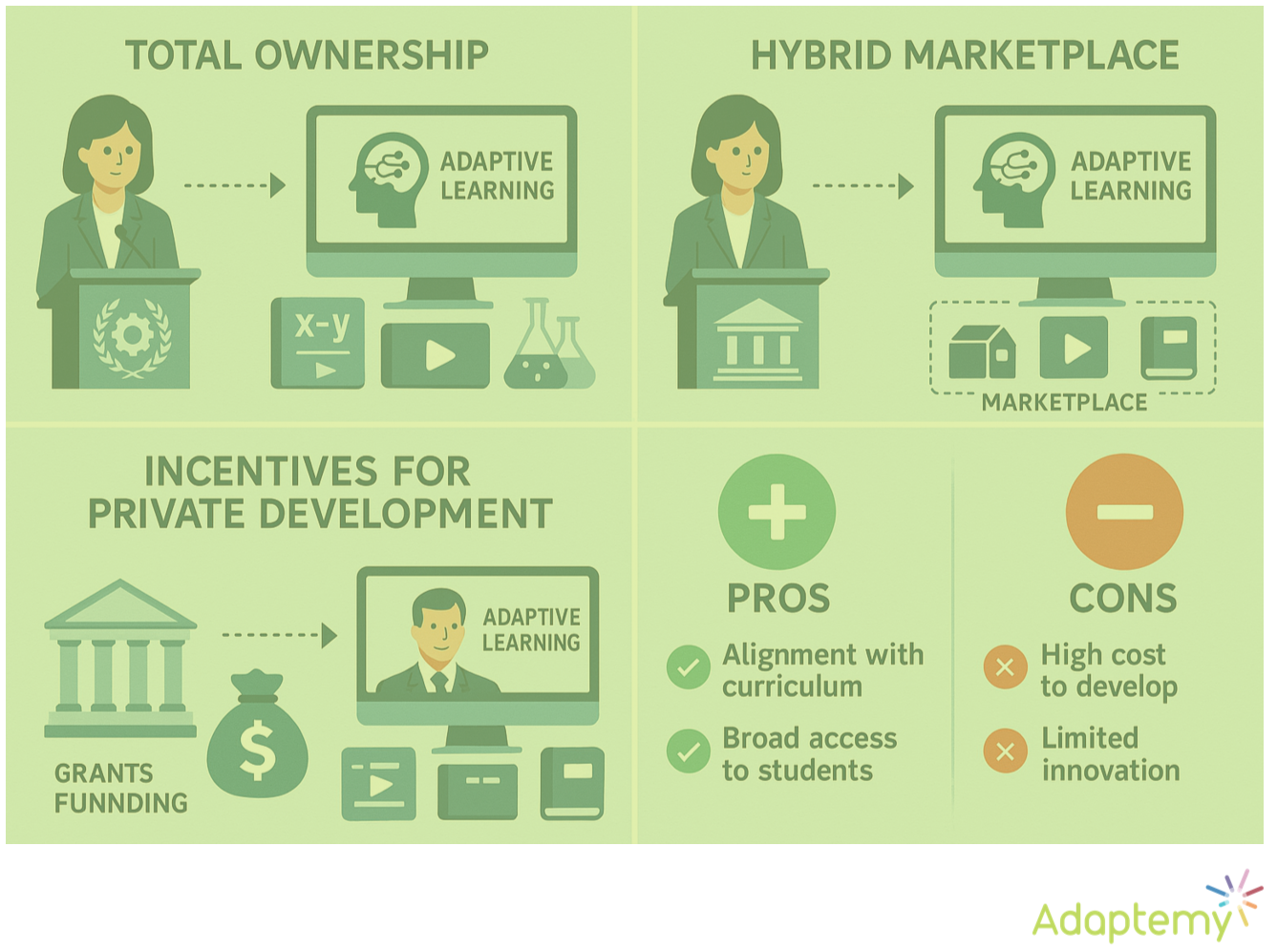
Adaptemy is actively engaged in supporting national AI education strategies through close collaboration with ministries of education and government-backed initiatives. From its work with the Ministry of Education in Singapore to align adaptive learning infrastructure with national curriculum goals, to its participation in projects across Europe that reflect the priorities outlined in Spain’s ENIA and Germany’s KMK resolution, Adaptemy consistently brings scalable, ethical, and pedagogically-sound adaptive learning solutions to the forefront.
In this blogpost, we will explore the three main models for Government Investment in Adaptive Learning and conclude with a focus on key markets where Adaptemy is already collaborating with GovTech to deliver impact for learners.
Introduction
Governments worldwide have recently adopted different approaches to implement adaptive learning (often AI-powered personalized learning) in K–12 and higher education. Below, we outline three common models, each with a recent example, noting the target education level and key features. We also highlight 1–2 pros and cons of each model based on these findings.
Model 1: Total Ownership – Government-Run Platform and Content
Under this model, the government builds and operates the adaptive learning platform and creates or controls the content. This ensures alignment with national curricula and broad access.
Singapore’s Adaptive Learning System (ALS) (K–12): In 2023, Singapore’s Ministry of Education (MOE) launched an Adaptive Learning System integrated into its national online portal for schools. The MOE-developed ALS uses machine learning to offer customized learning recommendations based on each student’s responses, allowing students to learn at their own pace. For instance, the ALS tool in mathematics profiles students into different learning pathways according to their competency, giving real-time feedback to teachers on who needs help and who can advance. This initiative fits the Total Ownership model: the government created the platform (within the Singapore Student Learning Space portal) and the adaptive content for subjects like math and geography. Similarly, South Korea announced in 2023 that it will phase in “AI-driven digital textbooks” in subjects like math and English by 2025, pairing teachers with AI assistant tutors to provide personalized learning adapted to student needs in public schools (More…). These illustrate governments directly developing AI-powered personalized learning tools for nationwide use.
Pros: This model guarantees that adaptive platforms align with the official curriculum and standards. It can promote equity by offering AI-powered personalized learning to all students as a public service, including support in local languages or specific subjects. There is also greater control over data privacy and content quality since the system is government-run.
Cons: It requires significant government investment and technical capacity to build and maintain advanced adaptive systems. Innovation may be slower or less diverse, as a single government platform might not evolve as rapidly as the private EdTech sector. The lack of competition might stifle external content development and limit teacher choice.
Model 2: Hybrid Marketplace – Government Platform with Private Content Providers
In this model, the government provides a central adaptive learning platform or portal, but partners with private publishers/EdTech companies who offer content (often courses or modules) through it. The government may vet or incentivize content, and often subsidizes access for learners.
India’s NEAT Platform (Higher Education): The National Educational Alliance for Technology (NEAT) is a government-approved marketplace platform launched in 2019 and expanded through “NEAT 3.0” in January 2022 (More…).
Operated by India’s Ministry of Education and AICTE, NEAT aggregates the “best-developed ed-tech solutions and courses” from 58 Indian and global start-up companies on a single platform. These solutions explicitly use artificial intelligence for a personalised and customised learning experience to improve student outcomes. NEAT focuses on university students and lifelong learners (enhancing employability skills), and the government distributes free course coupons to disadvantaged students. The private vendors provide adaptive learning content (for example, AI-powered courses in STEM, test prep, etc.), while the government provides the marketplace infrastructure and outreach. This Hybrid Marketplace approach lets the government curate AI-driven personalized learning offerings from the private sector on a national platform.
Pros: A hybrid marketplace leverages private-sector innovation and content diversity while giving the government oversight. Students and teachers benefit from a one-stop platform with a wide selection of AI-powered learning content and courses. The model can stimulate the local EdTech industry (as seen with dozens of start-ups on NEAT) and allow competition to improve quality. Governments can negotiate discounts or free seats (as India did, giving free access to many economically disadvantaged students) to bridge the digital divide. It combines public reach with private creativity.
Cons: Managing quality control is a challenge – the government must vet content and ensure it aligns with educational standards. There is a risk of uneven quality or coverage, as private publishers may focus on popular or profitable subjects, potentially leaving gaps in niche areas. Also, reliance on private content means sustainability isn’t fully in government hands – if companies withdraw or change business models, the content could disappear. Finally, integrating multiple external systems into one platform can be technically complex, and schools may need to handle different licenses or support issues for each provider.
Model 3: Incentivized Private Development – Grants/Funding to Build Adaptive Systems
Here, governments fund or incentivize companies and institutions to develop or enhance adaptive learning technologies, rather than building the tools themselves. This support can come via grants, innovation challenges, or research partnerships, and it often aims to spur new solutions or expand successful products to more users.
U.S. and Australia Funding AI-Adaptive EdTech (K–12): The United States has recently directed substantial funding toward AI-driven personalized learning tools. For example, in 2024 the U.S. federal government allocated about $190 million under the Every Student Succeeds Act specifically for scaling up AI-driven educational software in schools (More…).
This funding helps school districts procure or implement adaptive learning systems and other AI-powered tools. The Department of Education’s innovation programs (like the Education Innovation and Research grants) also support pilots of evidence-based personalized learning innovations in K–12 (More…). Meanwhile, in Australia, a joint federal-state initiative in 2023 provided AUD $4.7 million to pilot AI in education across eight Western Australian schools This pilot includes using AI to personalize aspects of learning or reduce teacher workload (e.g. automated lesson planning), demonstrating government support for integrating adaptive tech in classrooms. Another example is India’s 2023 budget, which set up Centers of Excellence in AI that encourage top universities and industry partners to collaborate on “innovative applications and scalable solutions” in education, among other fields. All these illustrate governments funding private or semi-private entities (companies, start-ups, or research consortia) to create and improve adaptive learning systems.
Pros: Funding programs spur innovation by leveraging private sector and academic expertise. Grants and R&D support can lead to new adaptive learning solutions or improvements in existing platforms without the government having to build from scratch. This model can bring in multiple solutions (for different needs) and drive competition. It also helps share the financial risk – companies get support to develop tools that might benefit public education, and successful tools can then be adopted at scale. In short, the government sets goals and provides resources, and the market responds with creative, AI-powered educational technologies.
Cons: The government has less direct control over the final products. Outcomes depend on the private recipients’ performance – some projects may fail or not align perfectly with curriculum needs. There can be fragmentation: if many different adaptive tools are developed via separate grants, schools might face a patchwork of solutions rather than one coherent system. Additionally, sustaining these innovations can be an issue: initial grants may produce a tool, but continued operation might require commercial viability or further funding, which isn’t guaranteed. Finally, ensuring equity can be harder – without careful policy, wealthy schools might adopt funded innovations faster, widening gaps unless the government also invests in deployment and training for all schools.
England (UK)
Policy Document: AI Barometer – Education – Centre for Data Ethics and Innovation (CDEI)
- Adaptive Learning Focus: Highlights Adaptive Learning Platforms (ALPs) and Intelligent Tutoring Systems as emerging AI tools to personalize learning paths for students. These systems use real-time data (e.g. student performance) to adjust content and provide tailored feedback, aiming to improve individual learning outcomes.
- Personalization & Teacher Support: Emphasizes that AI-driven adaptive learning can augment teaching – “teacher-augmentative personalised learning support” – rather than replace teachers. By offloading routine tasks (like grading or analytics), such tools free teachers to focus on pedagogy and one-on-one support.
- Opportunities vs. Challenges: England’s strong EdTech sector provides a base for AI adoption, but uptake is slowed by low educator trust and implementation barriers. The document notes concerns about data privacy and algorithmic bias as key issues to address so that adaptive learning technologies can be integrated safely and equitably.
“Significant progress has occurred in the field of Adaptive Learning Platforms (ALPs) and Intelligent Tutoring Systems (ITSs). These claim to offer a highly personalised learning experience, generating recommendations for learning materials and pathways through predictive analytics and real-time feedback.”
Key Risk/Issue: The report warns that lack of trust and data privacy concerns can hinder the use of AI-driven adaptive learning tools in classrooms. Educators worry about student privacy and potential bias in AI systems, which may slow adoption despite the potential benefits. Ensuring ethical, transparent AI use is therefore critical for these technologies to truly support personalized learning.
Singapore
Policy Document: Parliamentary Reply on Adaptive Learning Initiative – Ministry of Education
Adaptive Learning Program: The Ministry is developing an AI-enabled adaptive learning system as part of Singapore’s National AI Strategy. This system uses machine learning to gauge how each student responds to materials and activities, then customises the pace and content to suit individual learning needs. The goal is to enhance learning outcomes, especially for lower-progress learners, through personalization.
Integration & Teacher Role: The adaptive system will be integrated into Singapore’s national online learning platform (SLS) for broad access. Notably, the policy underscores that the teacher remains central – the AI tools “complement the role of the teacher” – addressing the issue that technology should assist, not replace, educators. Infrastructure upgrades (platform integration and devices) are pursued to support this blended approach.
“Developing an AI-enabled adaptive learning system to support teaching and learning in our schools is part of MOE’s plans under Singapore’s National AI Strategy. This approach complements the role of the teacher, who remains central to the learning experience. The system will use machine learning to tell how each student responds to learning materials and activities, and customise learning for each student.”
The initiative implicitly recognizes a risk of over-reliance on AI vs. human teachers. By explicitly stating that the teacher stays central in adaptive learning, the policy addresses the concern that AI might undermine the teacher’s role. Additionally, ensuring the necessary infrastructure and training is an issue: the system must be accessible through the SLS for all schools, which entails technical readiness and professional development so that no student or teacher is left behind in using the new AI tools.
Spain
Policy Document: Estrategia Nacional de Inteligencia Artificial (ENIA) – Government of Spain
AI in Education Focus: Spain’s national AI strategy includes education as a key area, calling for initiatives that leverage AI to personalize learning. It emphasizes addressing “viejos retos y nuevos desafíos en Educación, impulsando el aprendizaje personalizado” (“old and new challenges in education by promoting personalized learning”). This reflects a commitment to use AI (like adaptive learning systems) to tailor instruction to student needs and abilities.
- Talent and Training: ENIA highlights developing digital talent and AI skills through the education system. Alongside deploying AI for adaptive learning, it proposes boosting AI-related training in universities and vocational programs. This dual approach — AI in education (as a tool for learning) and AI for education (training the workforce) — is meant to modernize teaching methods and prepare citizens for an AI-driven society.
- Inclusion and Equity: A significant issue addressed is the digital divide. The strategy notes the need to reduce gaps in digital access and competencies across regions and social groups. It stresses ensuring schools are well-equipped with technology and connectivity so that adaptive learning innovations benefit all students. Equity is central: AI-powered personalized learning should be used to support disadvantaged learners and not widen existing educational inequalities.
“The strategy underscores “old challenges and new challenges in education, promoting personalized learning.On AI in Spanish education: “Spain has a higher-than-average percentage of schools highly equipped with technology.”
The ENIA identifies the digital divide and varying capacity of schools as a key issue. It calls out that not all educational institutions are equally prepared to implement AI-driven adaptive learning. Thus, a major focus is on investing in infrastructure and teacher training across the country to ensure that personalized/adaptive learning via AI can be deployed broadly without leaving certain regions or groups behind. Bridging skill gaps and providing equitable access to technology is crucial so that AI benefits all students, not just those in well-resourced settings.
Germany
Policy Document: “Handlungsempfehlung zum Umgang mit Künstlicher Intelligenz” – Resolution of the Bildungsministerkonferenz (Education Ministers Conference), Oct 2024. (Joint recommendation on AI use in schools, agreed by federal and state education ministers.)
- Adaptive Learning Systems in Schools: The policy explicitly recognizes adaptive learning systems as a significant innovation for German schools. It highlights that “intelligente, tutorielle Systeme und adaptive Lernsysteme in Schule [sind] genauso bedeutend” – intelligent tutoring systems and adaptive learning systems in schools are equally important (as other AI applications). These AI-powered systems can individualize the learning process, adjust to each student’s performance in real time, and thus support differentiated instruction in the classroom.
- Strategic Collaboration: The ministers stress that developing such AI tools (e.g. adaptive learning platforms, automated assessment tools) is resource-intensive and beyond the capacity of any single German state. They call for common standards and close cooperation across states (Länder) so that effective adaptive learning technologies can be scaled nationwide. A coordinated approach will also address data security and quality requirements consistently.
- Teacher Training and Critical Use: The recommendation underlines the need for a “constructive-critical” approach to AI in education. Schools should harness AI’s benefits for personalized learning and reducing teacher workload, while also teaching students about AI’s limitations (e.g. generative AI’s effects on information). Teachers and school leaders are urged to receive training on AI tools. This ensures that adaptive learning systems are implemented responsibly – enhancing learning personalization, but with educators skilled in guiding their use and aware of risks like algorithmic bias or over-reliance on automation.
Quotations (Adaptive Learning):
„AI in schools is a major innovation topic… Equally important are intelligent tutoring systems and adaptive learning systems in schools. That is why common standards and close cooperation in the Education Ministers Conference are so important.” – on jointly developing AI/adaptive learning tools)
Key Risk/Issue: A key issue noted is the need for unified action and standards to safely deploy AI in learning. German ministers point out that without collaboration, individual states may struggle with the technical, legal, and financial challenges of AI systems. Implicit risks include data privacy and quality control – hence the push for common standards. There is also an underlying concern about ensuring teachers and students use AI critically. The policy addresses this by recommending educator training and a critical perspective so that adaptive learning technologies are used to benefit learning, without undermining teaching quality or student data privacy.
Each of these documents underscores that “Adaptive Learning” with AI is seen as a promising tool to personalize education, while also highlighting the importance of teacher involvement, equity, data privacy, and collaborative governance as critical factors for successful implementation. The policies reflect a balance of optimism about AI’s potential to transform learning and caution to manage its risks in educational settings.