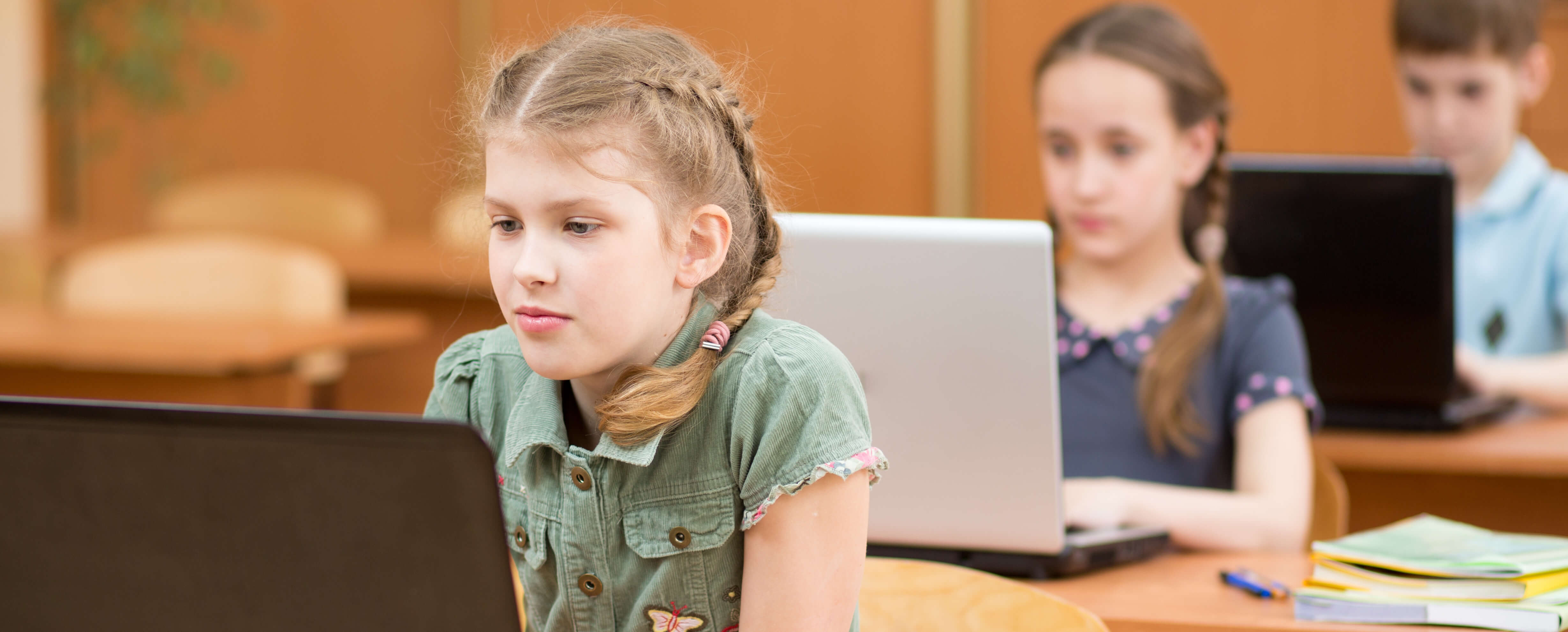
Finding effective learning paths and useful content that pushes each learner to their potential is not a straightforward task.
By integrating learning applications with Adaptemy’s AI engine, publishers are able to offer learners a more adaptive learning experience where they receive effective learning path recommendations and useful materials while maintaining their autonomy.
Guided by the learner model, content model and curriculum model, Adaptemy’s AI engine gives “best fit” individualised learning paths for learners with different characteristics. Here’s how.
Integrating Adaptemy’s AI Engine
Integrating Adaptemy’s AI engine into a learning application enables the application to provide recommendations to students.
The aim is to both present the students with specific goals and to prepare them for the given sessions. To promote student autonomy, the learning application can also allow learners to reject the given recommendations and to select themselves the concept to work with.
Recommendations have three parts:
- a specific concept to study
- a specific action (i.e. learn, attempt, revise, practice)
- tailored encouragements
How the Recommendations Work
At the centre of the recommendation is the specific concept to work with. The recommendation is given based on the updated learner model of the student at the time before a lesson is started, and the content and curriculum models.
Information from the learner model includes learner ability and previous learning experiences with each concept, as well as learner affective and motivational states. The engine considers which concepts have been worked on as well as the positions of the concepts in the knowledge graph of the course map.
The engine makes use of the curriculum model and learner model to identify concepts that are misconceptions for students and concepts that would have a high prerequisite activation in their memory.
The engine aims to maximise the learning gain in the student’s next lesson and prepare the student for more advanced concepts while not making the work too demotivational.
How Recommendations from the AI Engine are Integrated into a Learning Application
In the presented learning application below, there are three recommendation strategies available for students and directed by teachers.
In the first strategy the teacher does not provide input and the recommendation is done by the engine’s judgment.
In the second strategy, the teacher provides loose input. They do this by inputting the topic on which students should work and the number of concepts they should work.
In the third strategy, the teacher overwrites the engine’s recommendations and provides the learners with specific concepts through assignments.
In the first two strategies the learning application make use of the recommendations provided by the AI engine using a hybrid knowledge-based recommender algorithm.
The algorithm’s approach is to reduce misconceptions, increase coverage and to increase engagement by keeping students in flow. In the first strategy, it takes all concepts into consideration, while in the second strategy, it will filter and use only the concepts from the recommended topic.
When the teacher overwrites the engine’s recommendations, the student will receive the concept recommended by the teacher and the engine’s recommendation will be logged for offline analysis.
Details regarding the learning effectiveness of recommendations powered by Adaptemy’s AI engine can be found here. The results have shown that both learning success rate and improvement per lesson are higher if the engine-based recommendations are followed, in all the three cases.