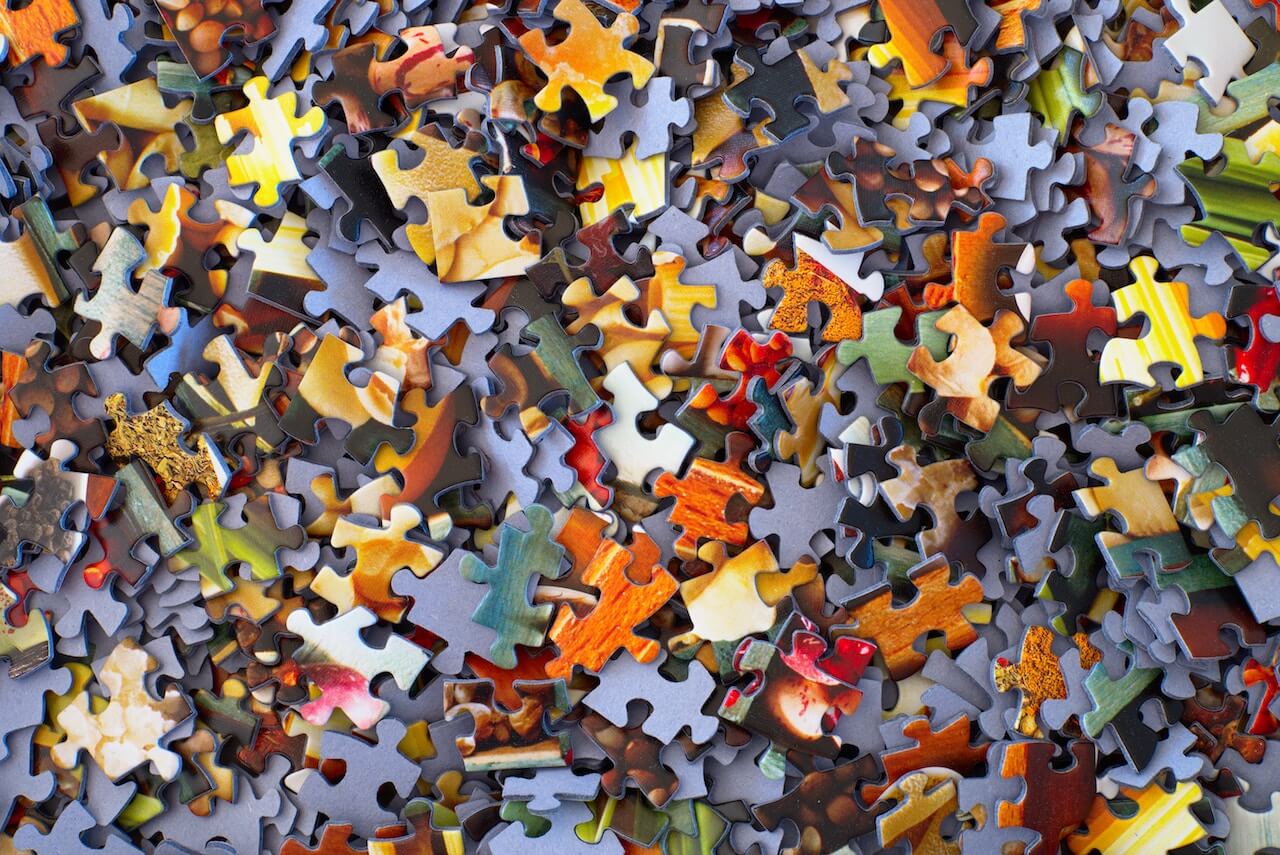
When content is used to orchestrate and personalise the learning experience it is vital to have an accurate model of the content. It is also important to automatically and intelligently update that model.
At Adaptemy, we use AI to do this, harnessing its insight to serve multiple purposes. This is how we update the content model.
What do we mean by the content model?
The content model contains all the metadata about the content such as: difficulty level, discriminance, probability of guessing, probability of having a slip, expected time to solve the question. It also contains real-time analytics of its usage and impact on learning effectiveness.
This rich information enables the AI adaptive learning engine to personalise the learning loop and accurately update the learner model.
The content model is also responsible for providing recommendations to publishers regarding what content needs to be improved. This information is learned and updated regularly by the AI adaptive learning engine based on usage and learning patterns.
How do we update the content model?
Collaborative Filtering and Item Response Theory are the underlying foundations of the entire system. The AI adaptive learning engine uses a hybrid algorithm of these theories to update its understanding of individual content items.
It does this through various metrics such as difficulty level, discriminance, probability of guessing, probability of having a slip and expected time to solve the question.
Some alternative adaptive learning engines are less complex in their consideration of updating the content model. They don’t assume each student has a unique capability level (or unique profile). Rather, they assume each student is a random sample within a population whose ability level on a concept follows a near-normal distribution.
The algorithm begins to pay dividends when data is available from 200 active users. Once the question metrics are estimated, the algorithm performs hierarchical clustering to optimise difficulty spread where specific difficulty buckets are needed.
Once the content model is updated, extensive recommendations for content improvement are available to the publisher. These might include recommendations to:
- improve questions that contain errors, confusions or have no power in measuring student ability
- re-categorise questions into new difficulty levels
- introduce new questions in a concept at the given difficulty level
- validate the association of a question to a concept
Our adaptive learning and AI experts are on hand to explain in detail how our engine works and how it benefits publishers. To find out more please get in touch.