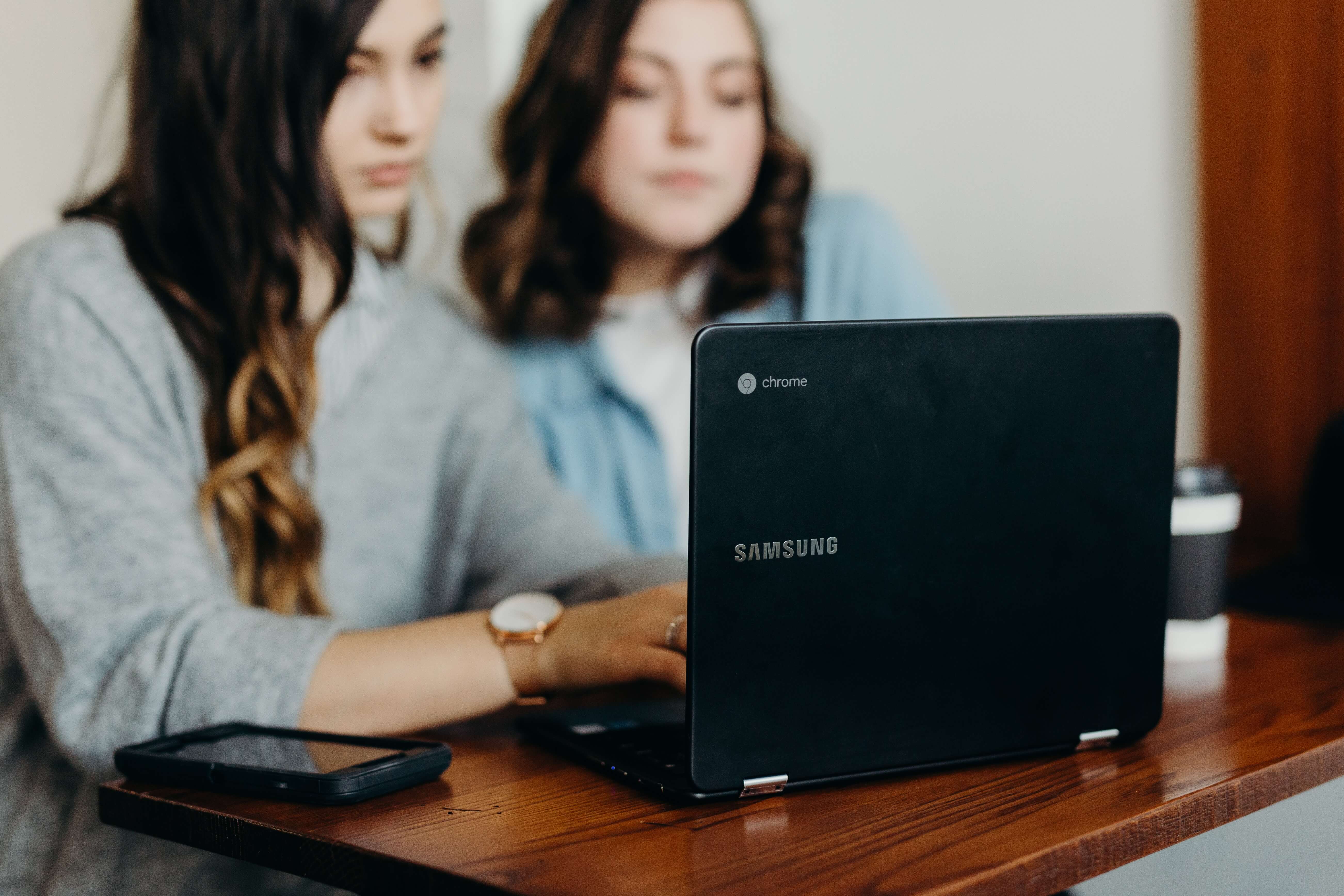
Understanding student ability is critical to the effectiveness of an adaptive learning solution.
By estimating what a student does or doesn’t know, the engine is able to present not just the next question in sequence but to create an extended learner model and to personalise the learning journey, maximising the student’s learning experience and potential.
Making use of ability estimates together with other inputs, a series of algorithms combine to enhance the learner model with additional information. This includes students’ attitudes towards learning, misconceptions and their affective and motivational state.
Here’s how the Adaptemy engine tracks and measure student ability
The Adaptemy engine follows the classical architecture of an adaptive and intelligent e-learning system and makes a separation between the curriculum model, content model, user model and the AI adaptation engine.
The curriculum model includes concepts and the relationships between them. The list of prerequisite links allows for misconception detections, a complex user model and enables multiple layers of personalisation and adaptation.
The content model contains all the metadata about the content and up-to-date analytics. The rich information from the content model enables the AI engine to personalise the learning loop and to accurately update the user model. For example, each question has information attached to it, such as the difficulty level and the quality of the question.
Students learn via Adaptemy by doing lessons, each of which is on a single concept consisting of a group of questions.
For each student, the Adaptemy engine maintains an ability profile on all the concepts in the curriculum. This is updated according to lesson outcomes.
As a student finishes a lesson, the ability profile of the concept that has been worked is updated based on direct evidence using a customised Item Response Theory (IRT). The customised IRT makes a distinction between previous evidence and the most recent evidence. It accounts for the most proper and recent information about the user.
The profile of the other concepts is also updated based on the lesson outcome as indirect evidence through Bayesian Networks updates. As there is empirical evidence that knowledge depreciation (forgetting) occurs, the engine models forgetting (what was forgotten after it is initially learned for each concept) and updates the student profile overnight.
Additionally, the user model is enhanced with information about previous work and behaviour.
The AI adaptation engine is responsible for updating the three models (user, content and curriculum) and for performing the adaptivity across various layers such as content difficulty adjustment, learning loops, motivation detection and learning path recommendations.
Join the discussion One Comment